What is explainable AI?
Table of Content
This article emphasizes the importance of explainable AI (XAI) in the adoption of AI systems. A hypothetical scenario is presented in which a high-accuracy AI model is developed to improve worker efficiency and safety in a steel tubing production line. However, the workers do not trust the system due to a lack of explainability, highlighting the crucial role of XAI in technology adoption.
The article provides an overview of XAI, its strengths, and limitations. XAI is crucial in addressing ethical concerns and developing trustworthy AI systems by enabling end-users to understand how the systems make decisions, particularly in high-stakes situations.
The Basics of Explainable AI
This article discusses the importance of explainable AI (XAI) in enabling human users to comprehend and trust the outputs generated by machine learning algorithms. Despite the abundance of research on explainability, there is still no widely accepted definition of XAI. However, leaders in academia, industry, and government have recognized the benefits of XAI and developed algorithms to address diverse contexts, such as healthcare and finance.
XAI is seen as a necessity for AI clinical decision support systems as it facilitates shared decision-making between medical professionals and patients and provides transparency. Explanations can take various forms depending on the context and purpose, as shown in Figure 1, which presents human-language and heat-map explanations of model actions for a hip fracture detection model designed for use by doctors. The Generated report helps doctors understand the model’s diagnosis and provides them with an easily understandable and vetted explanation.
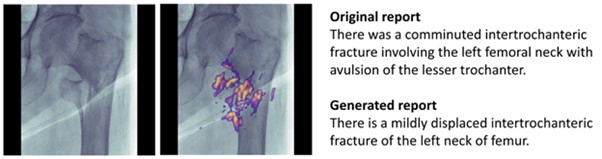
The visualization in Figure 2, presents an interactive and technical representation of the layers of a neural network, allowing users to experiment with the architecture of a neural network and observe how individual neurons evolve during the training process. Heat-map explanations are essential for understanding the inner workings of opaque models and developing better, more accurate models.
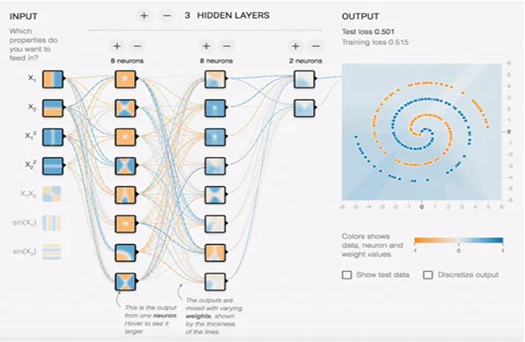
Figure 3, exhibits a graph created by the tool showcasing the connection between two inference score types. This interactive visualization enables users to evaluate model performance, pinpoint input attributes with the most significant impact on model decisions, and investigate data for biases or outliers. The What-If Tool plays a crucial role in enabling ML practitioners to better understand and communicate the results of their models, making machine learning more accessible and transparent for all.
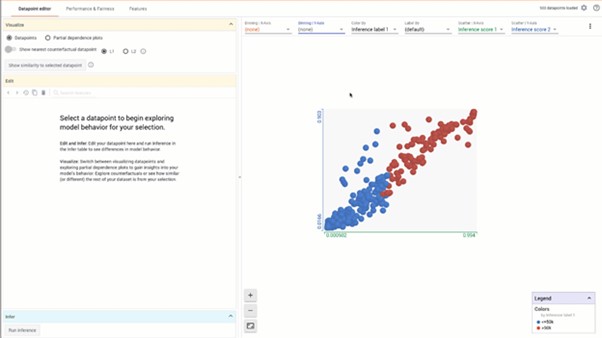
Developers and machine learning (ML) practitioners use explanations to ensure that project requirements are met and to promote trust and transparency. Explanations also help non-technical users understand how AI systems function and address their concerns, promoting system monitoring and auditability.
Techniques for creating explainable AI have been developed and employed throughout all stages of the ML lifecycle. Pre-modeling techniques involve analyzing data to ensure that the model is trained on relevant and representative data, while explainable modeling techniques involve designing inherently interpretable models. Post-modeling techniques generate explanations for specific decisions or predictions made by the model. By incorporating explainability techniques into the ML lifecycle, practitioners can ensure that their models are transparent, trustworthy, and auditable, promoting ethical and responsible use and adoption of AI systems.
Why Interest in XAI is Exploding
The study by IBM highlights the significant performance improvements that can be achieved through XAI, with users of their platform experiencing a 15 to 30 percent increase in model accuracy and a 4.1 to 15.6-million-dollar increase in profits.
The increasing prevalence of AI systems and their potential consequences make transparency crucial, and it is critical to develop these systems using responsible AI (RAI) principles. Legal requirements for transparency are emerging, such as the European Union’s General Data Protection Regulation (GDPR) and the California Consumer Privacy Act (CCPA), driving the development of XAI to meet new demands and ensure adherence to these regulations.
Current Limitations of XAI
The definitions of explainability and interpretability vary across papers and contexts, and clear and precise definitions are necessary to establish a shared language for describing and studying XAI topics. While many papers propose new XAI techniques, there is little real-world guidance on how to choose, implement, and test these explanations to meet project requirements.
The worth of explainability is also debated, with some arguing that opaque models should be replaced entirely with inherently interpretable models, while others contend that opaque models should be assessed through rigorous testing. Human-centered XAI research posits that XAI must extend beyond technical transparency to include social transparency. By resolving these issues, XAI can provide insights into complex AI models and promote trust in these systems, benefiting both technical and non-technical stakeholders.
Why is the SEI Exploring XAI?
The US government recognizes the importance of explainability in establishing trust and transparency in AI systems. Deputy Defense Secretary Kathleen H. Hicks emphasized the need for commanders and operators to trust the legal, ethical, and moral foundations of explainable AI, and for the American people to trust the values integrated into every application.
The US Department of Defense has adopted ethical principles for AI, indicating an increasing demand for XAI in the government. The US Department of Health and Human Services also lists promoting ethical and trustworthy AI use and development, including explainable AI, as one of the focus areas of their AI strategy.
More info: insights.sei.cmu.edu